Batch Processing in SaaS: How It Works, Use Cases, Tools
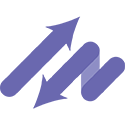

Batch processing in SaaS is a method for handling large volumes of data in groups rather than processing each piece individually. Here's what you need to know:
- Definition: Collecting data over time and processing it in predefined groups without user interaction
- Key benefits: Efficient handling of big data, cost savings, improved data quality
- Common use cases: Financial operations, data analysis, system maintenance
- How it works:
- Collect and prepare data
- Schedule and run jobs
- Handle errors
- Create and share results
Top batch processing tools for SaaS:
Tool | Best For | Key Feature |
---|---|---|
AWS Batch | Tech-savvy teams | Easy file sharing |
Azure Batch | Microsoft-centric businesses | Good scheduling |
Apache Airflow | Complex workflows | Large community |
Luigi | Smaller projects | Simple pipeline focus |
ActiveBatch | Cross-technology processes | Reliable task automation |
While batch processing offers many advantages, it can be slow and risks working with outdated data. The future of batch processing in SaaS involves cloud integration, AI/ML enhancements, and a blend of batch and real-time processing for optimal performance.
Related video from YouTube
2. Basics of Batch Processing in SaaS
2.1 Main Ideas
Batch processing in SaaS is a method of handling large amounts of data by grouping it into batches and processing them together. This approach is useful for tasks that don't need real-time results.
Key aspects of batch processing:
- Processes data in fixed intervals (e.g., daily, weekly)
- Handles complex transformations and analytics
- Works with minimal user interaction
- Improves efficiency for big data tasks
2.2 Batch vs. Real-Time Processing
Feature | Batch Processing | Real-Time Processing |
---|---|---|
Data Handling | Large volumes at once | Individual data points |
Processing Time | Scheduled intervals | Immediate |
Resource Usage | More efficient for big data | Can be resource-intensive |
Use Cases | Monthly reports, payroll | Live stock prices, fraud detection |
Latency | Higher (minutes to hours) | Lower (seconds or less) |
2.3 Parts of a Batch Processing System
A typical batch processing system in SaaS includes:
- Data Sources: Where the information comes from (e.g., databases, files)
- Data Storage: Where batches are kept before and after processing
- Processing Engine: The core component that runs the batch jobs
- Scheduling System: Manages when batches are processed
- Error Handling: Deals with issues during processing
- Output Management: Handles the results of batch jobs
For example, a SaaS company might use Google Cloud Platform for batch processing. They could use Google Cloud Storage for data storage, Apache Beam for building processing pipelines, and Google Cloud Composer for scheduling.
"Implementing thorough data quality controls is crucial. This involves setting up procedures to consistently verify accuracy and completeness, which maintains the integrity of your batch processing."
3. How Batch Processing Works in SaaS
3.1 Collecting and Preparing Data
Batch processing in SaaS starts with gathering data from various sources. This can include:
- Databases
- Files
- Sensors
- APIs
Once collected, the data needs to be prepared. This involves:
- Cleaning: Removing errors or inconsistencies
- Validating: Ensuring data meets required standards
- Transforming: Converting data into a suitable format for processing
For example, a SaaS company might use SQL queries to pull customer data from a database, then clean it by removing duplicate entries and standardizing formats.
3.2 Scheduling and Running Jobs
After data preparation, the next step is scheduling and running batch jobs. This process typically involves:
- Job Definition: Specifying what tasks need to be done
- Scheduling: Setting when jobs should run (e.g., daily, weekly, monthly)
- Resource Allocation: Assigning computing resources to jobs
- Execution: Running the jobs according to the schedule
Many SaaS companies use tools like Apache Airflow or AWS Batch for job scheduling and execution. These tools help manage complex workflows and ensure jobs run efficiently.
3.3 Handling Errors
Error handling is a key part of batch processing. Common approaches include:
Error Handling Method | Description |
---|---|
Retry Mechanisms | Automatically attempt to rerun failed tasks |
Logging | Record detailed information about errors for analysis |
Alerting | Notify administrators of critical failures |
Graceful Degradation | Continue processing other parts of the batch if one part fails |
For instance, if a data import task fails, the system might retry the import three times before sending an alert to the operations team.
3.4 Creating and Sharing Results
The final stage involves generating outputs and distributing them. This can include:
- Creating reports
- Updating databases
- Sending notifications
- Triggering other processes
Results are often shared through:
- Dashboards
- Email reports
- API endpoints
- File exports
For example, after processing monthly sales data, a SaaS platform might generate PDF reports for each client and automatically email them to the respective account managers.
"Batch processing is a cost-effective means of handling large amounts of data at once."
4. Benefits of Batch Processing in SaaS
Batch processing offers several key advantages for SaaS companies:
4.1 Handling Big Data
Batch processing excels at managing large volumes of data:
- Processes vast amounts of information efficiently
- Ideal for tasks like data warehousing and ETL operations
- Handles complex computations on extensive datasets
For example, Netflix uses batch processing to handle 450 billion unique events daily from over 100 million members globally.
4.2 Saving Money
Batch processing can reduce costs for SaaS companies:
Cost-Saving Aspect | Description |
---|---|
Resource Optimization | Runs during off-peak hours, using idle computing power |
Reduced Infrastructure | Requires fewer real-time processing resources |
Efficient Data Handling | Processes large volumes of data in a single run |
4.3 Better Data Quality
Batch processing improves data accuracy:
- Allows for thorough data validation and cleansing
- Provides time for complex data transformations
- Enables consistent application of business rules across large datasets
4.4 Using Resources Well
Batch processing optimizes resource usage:
- Schedules jobs when system load is low
- Balances workloads across available computing resources
- Maximizes throughput for data-intensive tasks
For instance, many companies run batch processes for financial statement generation during off-hours, making efficient use of system resources without impacting daily operations.
5. Problems with Batch Processing
While batch processing offers many benefits, it also comes with its share of challenges. Let's explore the main issues SaaS companies face when implementing batch processing systems.
5.1 Slow Processing Times
Batch processing can be slower than real-time options, especially when dealing with large volumes of data. This can lead to:
- Delayed insights and decision-making
- Potential bottlenecks in data pipelines
- Increased resource usage during processing
To address this, many companies schedule batch jobs during off-peak hours. For example, Netflix processes 450 billion unique events daily from over 100 million members globally, running most of these jobs overnight to minimize impact on user experience.
5.2 Managing Big Jobs
Handling large, complex batch jobs can be challenging for SaaS companies. Common issues include:
Challenge | Description |
---|---|
Resource allocation | Ensuring enough computing power and memory for big jobs |
Job scheduling | Managing dependencies and avoiding conflicts between jobs |
Error handling | Dealing with failures in long-running processes |
Thomas Oppong, Founder at Alltopstartups, notes:
"Big businesses employ batch processing technologies to complete big job orders effectively. For instance, banks, healthcare facilities, and accounting firms use batch processing to generate reports and process transactions."
To tackle these challenges, companies often use workflow management tools like Apache Airflow or Apache Oozie. These tools help manage dependencies, handle retries, and recover from failures seamlessly.
5.3 Old Data Risk
One of the biggest drawbacks of batch processing is the risk of working with outdated data. This can happen due to:
- Long intervals between batch runs
- Processing delays caused by large data volumes
- System failures or errors during processing
For SaaS companies dealing with time-sensitive data, this can be a major concern. For example, a financial services company using batch processing for transaction analysis might miss detecting fraudulent activities in real-time.
To mitigate this risk:
- Run batch jobs more frequently when possible
- Use a mix of batch and real-time processing for critical data
- Implement robust error handling and recovery mechanisms
sbb-itb-96038d7
6. Where Batch Processing is Used in SaaS
Batch processing is a key feature in many SaaS applications. Let's look at how different industries use it:
6.1 Finance and Accounting
In finance, batch processing handles large volumes of transactions efficiently. For example:
- Payroll: Companies use batch processing to generate payslips and handle tax submissions.
- Bank Transactions: Banks process end-of-day transactions in batches.
"Batch processing is a cost-effective means of handling large amounts of data at once."
6.2 Data Storage and Analysis
Data-heavy industries rely on batch processing for:
- Data Warehousing: Moving large datasets into storage systems.
- Business Intelligence: Running complex queries on big data.
6.3 Customer Management
SaaS companies use batch processing to manage customer data, including:
- Updating customer records
- Processing bulk orders
- Generating customer reports
6.4 Supply Chain
In supply chain management, batch processing helps with:
- Inventory updates
- Order processing
- Shipping logistics
6.5 HR and Payroll
HR departments use batch processing for:
- Timesheet processing
- Benefits calculations
- Tax form generation
Industry | Batch Processing Use Case |
---|---|
Finance | Payroll, bank transactions |
Data | Data warehousing, business intelligence |
Customer | Bulk updates, report generation |
Supply Chain | Inventory management, order processing |
HR | Timesheet processing, benefits calculations |
Batch processing in SaaS offers speed, accuracy, and cost savings. It's particularly useful for tasks that don't need real-time processing.
"BrightPay's batch operation features enable bureaus to save time on manual administrative tasks, especially where payrolls don't change from week to week or where a large number of single-director companies are on the payroll software."
7. Batch Processing Tools for SaaS
SaaS companies need strong batch processing tools to handle large data volumes efficiently. Let's look at some top options:
7.1 Top Batch Processing Tools
-
AWS Batch: Amazon's cloud-based service for running batch computing jobs. It's great for handling complex workloads and scaling resources automatically.
-
Azure Batch: Microsoft's tool for running large-scale parallel and batch computing jobs. It's well-suited for businesses already using Azure cloud services.
-
Apache Airflow: An open-source platform to program workflows. It's popular for its large community and wide range of features.
-
Luigi: Spotify's tool for building complex pipelines of batch jobs. It's simpler than Airflow but lacks some advanced features.
-
ActiveBatch: A comprehensive tool that offers task execution automation and integrates well with various technologies.
7.2 Tool Comparison
Tool | Pros | Cons | Best For |
---|---|---|---|
AWS Batch | Easy file sharing, public/private options | Slow dashboards, weak documentation | Tech-savvy teams |
Azure Batch | Easy scripting, good scheduling | UI could be clearer | Microsoft-centric businesses |
Apache Airflow | Large community, many features | Steep learning curve | Complex workflows |
Luigi | Simple, focused on pipelines | Limited scalability | Smaller projects |
ActiveBatch | Reliable task automation | Pricing based on environments | Cross-technology processes |
7.3 Working with Other SaaS Tools
Batch processing tools often need to work with other SaaS systems. Here's how some tools connect:
- AWS Batch: Works well with other AWS services like S3 for storage and Lambda for serverless computing.
- Azure Batch: Integrates smoothly with Azure's ecosystem, including Azure Storage and Azure Functions.
- Apache Airflow: Offers many plugins to connect with various SaaS tools, from databases to messaging services.
- Luigi: Can work with Hadoop, Spark, and other big data tools, though with less built-in support than Airflow.
- ActiveBatch: Provides integrations with many business applications and databases.
When picking a batch processing tool, think about your team's skills, your current tech stack, and how well the tool fits your specific needs. Test a few options to find the best fit for your SaaS company.
8. Tips for Using Batch Processing in SaaS
8.1 Creating Good Batch Jobs
To make batch jobs work well in SaaS:
- Define clear goals for each job
- Pick the right data and processing method
- Break big jobs into smaller parts
- Test jobs thoroughly before running them
8.2 Improving Speed and Growth
Make batch processing faster and more scalable:
- Use cloud computing for flexible resources
- Try distributed computing to split work across machines
- Optimize database queries and data access
- Use caching to speed up repeated operations
8.3 Keeping Data Safe
Protect your data during batch processing:
- Encrypt sensitive data at rest and in transit
- Use access controls to limit who can run jobs
- Back up data regularly
- Follow data privacy laws and rules
8.4 Watching and Recording
Keep an eye on batch processing:
Monitoring Aspect | Why It's Important | How to Do It |
---|---|---|
Job status | Catch issues early | Use real-time dashboards |
Resource use | Avoid overload | Set up alerts for high CPU/memory use |
Error rates | Find and fix problems | Log errors and review regularly |
Processing time | Spot slowdowns | Track job duration trends |
9. Future of Batch Processing in SaaS
9.1 Cloud Batch Processing
Cloud tech is changing how SaaS companies handle batch jobs. It lets them scale up or down as needed, cutting costs and boosting efficiency.
Cloud Batch Processing Benefits | Description |
---|---|
Scalability | Adjust resources based on workload |
Cost-effectiveness | Pay only for resources used |
Flexibility | Run jobs from anywhere, anytime |
Many SaaS firms now use cloud platforms for their batch tasks. This shift helps them handle big data sets without buying expensive hardware.
9.2 AI and Machine Learning
AI and ML are making batch processing smarter. They can:
- Spot patterns in data
- Predict outcomes
- Optimize job scheduling
For example, ML algorithms can figure out the best time to run batch jobs, cutting down on processing time and resource use.
9.3 Mixing Batch and Real-Time
SaaS companies are blending batch and real-time processing. This combo lets them:
- Handle big data sets (batch)
- Respond to urgent needs (real-time)
A good example is how some firms use batch processing for nightly reports, but switch to real-time for critical updates during the day.
As batch processing evolves, SaaS companies that adapt will have an edge. They'll be able to handle more data, faster, and make smarter choices based on that data.
10. Wrap-Up
Batch processing in SaaS has come a long way. It's now a key part of how many companies work with data. Here's what you need to know:
- Big data handling: Batch processing helps SaaS companies deal with large amounts of data at once.
- Cost savings: By running tasks in batches, companies can save money on computing resources.
- Data quality: Batch processing can help improve data quality by running checks and fixes on large datasets.
But it's not all smooth sailing. Batch processing can be slow, and managing big jobs can be tricky. There's also a risk of working with old data if batches aren't run often enough.
Here's a quick look at where batch processing is used in SaaS:
Industry | Use Case |
---|---|
Finance | End-of-day reconciliation |
HR | Payroll processing |
Supply Chain | Inventory updates |
Customer Management | Bulk email sends |
Tools are getting better too. Cloud-based options are making it easier and cheaper to run batch jobs. AI and machine learning are helping to make batch processing smarter and more efficient.
Looking ahead, we're seeing a mix of batch and real-time processing. This combo lets companies handle big data sets while still responding quickly when needed.
Remember, batch processing isn't just about running jobs overnight anymore. It's evolving to keep up with the fast pace of modern business. As one expert put it:
"The current work landscape is decentralized and operates in real time, involving unprecedented complexity in data processing."
For SaaS companies, getting batch processing right can mean the difference between drowning in data and surfing the wave of insights it can provide.
FAQs
What is the use case of batch processing?
Batch processing has many uses across different industries. Here's a quick look at some key applications:
Industry | Use Case |
---|---|
Finance | End-of-day calculations, transaction processing, statement generation |
HR | Payroll processing, tax calculations |
Supply Chain | Inventory updates, order processing |
Data Analysis | Trend identification, pattern recognition |
IT | Data backups, system updates |
For example, in the banking sector, batch processing is often used for nightly account reconciliations. Banks process thousands of transactions during the day, then run a batch job overnight to update all account balances.
In the realm of data analysis, companies use batch processing to mine large datasets for insights. This might involve analyzing customer behavior patterns or market trends over time.
Batch processing is also key in many SaaS applications. It allows companies to handle large volumes of data efficiently, often at off-peak hours to minimize system load.
For instance, a SaaS email marketing platform might use batch processing to send out millions of emails for a client's campaign. Instead of sending them all at once (which could overload the system), the emails are processed and sent in batches throughout the day.
Remember, batch processing is best for tasks that:
- Don't need real-time results
- Involve large amounts of data
- Can be automated
- Are done regularly (daily, weekly, monthly)
Related posts
Ready to get started?